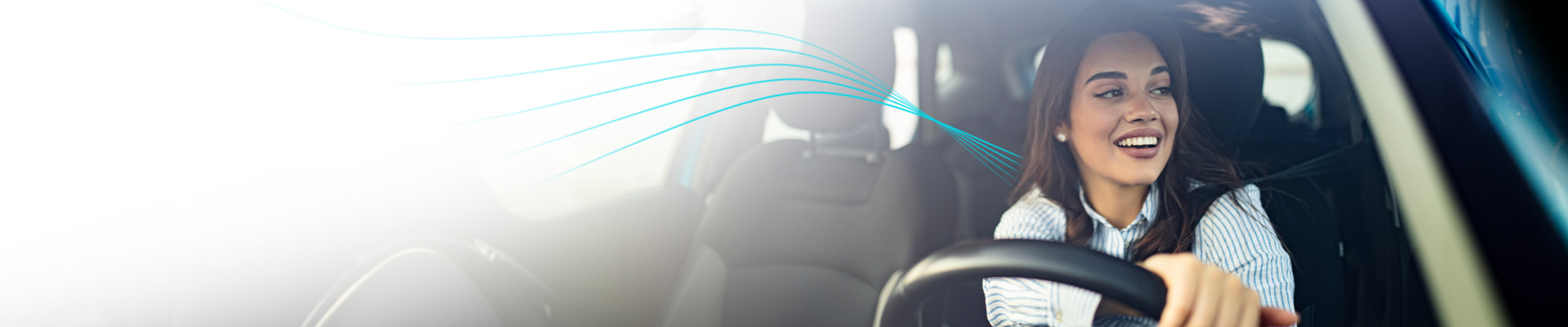
Driving Ahead of the Curve: How AI is Shaping Automotive Cybersecurity
The automotive industry sits at the intersection of some of today’s most transformative innovations. As vehicles become increasingly software-defined, connected, and automated, we are witnessing unprecedented advancements in comfort, safety, and user experience. Yet alongside these leaps forward come significant new challenges—particularly in the realm of cybersecurity.
Over the past decade, the digital infrastructure in modern vehicles has evolved from basic infotainment systems into complex networks comprising millions of lines of code and diverse computing platforms. Intelligent driver-assistance features, over-the-air software updates, and vehicle-to-everything (V2X) communications are reshaping what it means to drive a car. At the same time, these technological strides have created new opportunities for cybercriminals. The increasing frequency and sophistication of attacks on vehicles and their supply chains send a clear signal: the automotive sector must make cybersecurity a core pillar of its future.
A Changing Threat Landscape
In the last few years, we’ve seen a surge of automotive cyber incidents—from large-scale data breaches affecting corporate networks to high-profile demonstrations of remote vehicle takeovers. Whereas early concerns focused mainly on infotainment hacks or ransomware attacks on OEMs, today’s threat landscape extends much deeper. Attackers now have their sights set on critical driving functions, personal data, and, increasingly, on the evolving ecosystem of monetized vehicle capabilities.
As the industry moves into creating additional revenue streams—such as on-demand services and user-enabled capabilities—cybercriminals see new financial motives for their efforts. The prospect of hacking premium features, manipulating subscription-based services, or “jailbreaking” paid add-ons incentivizes attackers who seek to profit directly from these innovations. In this environment, cybersecurity threats no longer manifest purely as abstract code manipulations; they have a tangible economic dimension, where criminals can directly convert exploited vulnerabilities into revenue.
Compounding this risk is the extensive nature of the automotive supply chain. Most disruptions—and thus vulnerabilities—originate from indirect suppliers at the Tier 2 and Tier 3 levels. In an environment where a single compromised software library can ripple through multiple OEMs and millions of vehicles, transparency, trust, and continuous risk monitoring have never been more vital.
Adding to the complexity are forthcoming regulations such as UNECE R155 and R156, which require all new vehicles and their supply networks to meet rigorous automotive cybersecurity standards. Compliance is not optional; it’s fundamental to ensuring consumers maintain trust in these evolving platforms.
AI: A New Frontline in Cyber Defense
As attackers grow more sophisticated, defenders must respond in kind. Artificial intelligence (AI), widely recognized for its transformative potential in areas ranging from manufacturing optimization to predictive maintenance, is proving equally valuable in cybersecurity.
By leveraging advanced machine learning techniques, anomaly detection models, and predictive analytics, AI systems can rapidly sift through enormous volumes of vehicle and supply chain data, identifying suspicious activities long before they escalate into full-blown incidents. This capability is crucial in automotive contexts, where even minor anomalies can signal a potential intrusion with life-or-death implications.
Consider the scenario of real-time threat detection. AI-enabled solutions can continuously monitor vehicle networks, V2X communications, and firmware updates, recognizing when incoming data strays from the established norm. If, for example, a malicious actor attempts to introduce unauthorized commands into a vehicle’s safety-critical systems, AI models can instantly flag and isolate that intrusion—potentially mitigating an attack in progress.
AI also supports predictive analytics. By examining historic incident patterns, known vulnerabilities, and global threat intelligence feeds, AI helps organizations anticipate where cybercriminals may strike next. This forward-looking approach transforms cybersecurity from a reactive to a proactive discipline, enabling OEMs and their partners to patch vulnerabilities, refine architectures, and strengthen defenses long before an attacker even appears.
Building Proactive, Collaborative Ecosystems
The complexity of modern vehicles demands holistic, system-wide cybersecurity. No OEM or supplier can tackle these challenges alone. To fully capitalize on the potential of AI and next-generation security technologies, automotive stakeholders must embrace cooperation—exchanging threat intelligence, defining industry-wide best practices, and establishing transparent supplier relationships.
This collaboration may involve cross-industry consortia, shared threat databases, and standardized cybersecurity frameworks that raise the protective baseline for all participants. One possible alternative is to leverage solutions from vendors that can aggregate information and data from various customers and suppliers. By pooling insights into a collective knowledge base—one enriched with threat intelligence, patching best practices, and historical incident data—these solutions help the entire ecosystem learn and adapt more quickly.
Such vendor-driven data aggregation can streamline the detection of emerging attack patterns and vulnerabilities, distributing early warnings to all participants and enabling a more unified, rapid response. When a new exploit is discovered, the industry can coordinate, apply fixes, and close security gaps collectively, rather than leaving individual companies to face the threat in isolation.
At the heart of this collaborative framework is comprehensive supply chain visibility. By mapping suppliers across multiple tiers, continuously monitoring their cybersecurity postures, and deploying AI-driven tools to detect emerging threats, the industry can respond swiftly and decisively—no matter the threat’s origin.
Bridging Cyber and Safety Requirements
Automotive cybersecurity is not merely about protecting data. It’s also about safeguarding human lives. With the rise of advanced driver-assistance systems (ADAS) and the drive toward full autonomy, security failures can result in more than just financial or reputational damage—they can threaten passenger safety and public trust in autonomy itself.
This raises important considerations around how we integrate cybersecurity with functional safety. AI can serve as a connective tissue between these traditionally separate domains. For instance, when sensors feeding critical driver-assistance decisions start behaving unexpectedly—perhaps due to a cyber intrusion—an AI model can detect the anomaly and alert both security and safety systems to take action. The vehicle might temporarily revert to manual control or follow a defined safe-stop procedure, minimizing the risk to occupants and other road users.
As vehicles continue to evolve into intelligent, software-defined platforms, cybersecurity and safety functions will increasingly converge. AI’s ability to rapidly learn, adapt, and respond to ever-changing conditions makes it an ideal ally in this integrated environment. By weaving cybersecurity into the automotive DNA from the earliest design stages and continuously refining defenses post-deployment, the industry can keep pace with emerging threats without sacrificing performance or user experience.
The Dark Side of AI: Powering Advanced Cyber Attacks
While AI offers significant advantages for defenders, it also empowers cybercriminals with sophisticated tools for launching advanced attacks. Attackers are increasingly leveraging AI for automated vulnerability scanning, adaptive malware, and targeted phishing campaigns, creating new challenges for cyber security teams. For instance, AI-driven attackers can quickly uncover vulnerabilities in vehicle systems, such as unpatched firmware or outdated security protocols, through automated scanning. This rapid identification allows them to exploit weaknesses more efficiently, enabling quicker attacks on a vehicle’s ECU firmware by targeting known security flaws faster than traditional methods allow.
Adaptive malware is another rising threat. AI allows malware to adjust its behavior dynamically, depending on the environment in which it operates. For example, AI-enhanced malware targeting a vehicle’s infotainment system might behave normally during initial analysis but trigger malicious payloads once it detects its integration with the vehicle’s broader network.
Moreover, AI can be used to craft highly targeted phishing attacks. Generative AI models can personalize phishing emails to deceive employees within automotive organizations, making it harder for recipients to distinguish between legitimate messages and attacks. For example, an AI might generate phishing emails that appear to come from trusted partners or coworkers, increasing the likelihood of successful credential theft.
Positioning for the Future
This is a pivotal moment for the automotive sector. As the industry drives toward a future of connected, automated, and AI-powered vehicles, robust cybersecurity measures are essential. Companies that embrace next-generation technologies, foster cross-industry collaboration, and view cybersecurity through the lens of both product integrity and user safety will define the next chapter of automotive excellence.
At PlaxidityX (formerly Argus Cyber Security), we believe leadership in this space comes from looking beyond one-off solutions. It’s about adopting a forward-thinking vision that integrates cybersecurity into every step—from R&D and supply chain management to long-term maintenance and eventual decommissioning of vehicles. It’s about harnessing the power of AI to achieve not only smarter detection but also more strategic prevention. And it’s about anticipating future threats to stay one step ahead.
Cyber threats will only grow more sophisticated in the coming years. By proactively embedding cybersecurity into the automotive ecosystem and fully leveraging the potential of AI, we can secure a safer, more resilient future. The path forward demands innovation, vigilance, and a commitment to building trust. Those who rise to this challenge today will steer the automotive industry confidently into tomorrow.
Published: October 15th, 2024